AI Personalized Intelligence involves utilizing artificial intelligence (AI) technologies to customize experiences, products, or services according to individual preferences, behaviors, and requirements. This concept is revolutionizing multiple industries and some you may not even realize that you’re already experiencing. Here are some of the basics:
- Data Analysis: AI employs machine learning algorithms to examine extensive datasets, which encompass browsing history, purchasing patterns, social media engagement, and demographic details. This examination aids in comprehending and forecasting individual preferences.
- Personalized Recommendations: In the realm of e-commerce, artificial intelligence can recommend products or content tailored to previous user interactions, resulting in enhanced customer engagement and higher sales. Similarly, streaming platforms such as Netflix and Spotify leverage AI to create customized playlists and suggest movies.
- Customized Learning: In the field of education, artificial intelligence tailors learning experiences by customizing content and instructional approaches to align with each student’s unique learning preferences, ultimately improving educational results.
- Customer Interaction: AI-powered chatbots and virtual assistants deliver customized customer support by addressing inquiries in real-time, offering responses that are specifically adapted to the user’s past interactions or present situation.
- Healthcare: AI-driven personalization in healthcare has the potential to create customized treatment strategies that consider an individual’s genetic makeup, medical history, and lifestyle choices, ultimately striving for improved health results.
AI Personalized Intelligence Advantages
- Improved Engagement: Enhancing user engagement can be achieved by providing content or services that closely align with their interests.
- Higher Conversion Rates: Tailored recommendations often result in improved sales outcomes in retail settings and increased click-through rates in marketing campaigns.
- Enhanced Customer Satisfaction: When users receive personalized experiences, they are more likely to feel recognized and appreciated, which cultivates brand loyalty.
- Efficiency in Service Delivery: Implementing automated personalized responses not only conserves time and resources but can also enhance the overall quality of service.
However, there are challenges, including:
- Data Privacy: The widespread utilization of personal data brings about significant privacy issues. It is essential to maintain transparency and security in the management of this data.
- Bias and Accuracy: If the datasets employed for training AI models include biases, the resulting personalization may unintentionally perpetuate these biases.
- Ethical Concerns: Striking a balance between personalization and privacy invasion is critical and requires meticulous attention.
The movement towards AI Personalized Intelligence is on the rise, as organizations such as IBM, AWS, and OpenAI investigate or deploy solutions that enable AI to adapt based on individual user data, thereby delivering a tailored experience. This strategy is regarded not only as a technological innovation but also as a strategic initiative aimed at improving customer relationships and business results in a progressively digital landscape.
To learn more about how AI can help your business, Book An Appointment and we can share what we know to help you.
AI Personalized Intelligence Examples
To help understand this better, let’s look at where you are currently experiencing AI personalized intelligence. You may agree that it’s pretty helpful and it’s popularity is certainly on the rise.
E-Commerce
- Amazon: Employs artificial intelligence to generate sections such as “Customers who purchased this also purchased” and “Recommended for you,” customizing product suggestions according to your browsing and purchase history.
- Alibaba: Their AI framework evaluates user interactions to deliver tailored shopping experiences, featuring personalized search outcomes and product recommendations.
Streaming Services
- Netflix: Utilizes a recommendation system that proposes films and television series tailored to your viewing patterns and the preferences of viewers with similar tastes.
- Spotify: Offers customized playlists such as “Discover Weekly” and “Daily Mixes,” which are designed according to your listening preferences, the time of day, and your current mood.
Social Media
- TikTok: Employs an advanced algorithm to tailor the content feed, presenting videos that resonate with user interests and often anticipating what will capture their attention even before they realize it.
- Instagram: Curates your feed and explore section based on what it believes you’ll enjoy, taking into account your interactions, the duration of your engagement with posts, and various user data.
Healthcare
- IBM Watson Health: Employs AI technology to customize treatment strategies for cancer patients by examining their medical histories, genetic information, and the latest research to recommend the most suitable therapies.
- Fitbit: Tailors fitness and wellness suggestions according to your activity levels, sleep habits, and heart rate information.
Banking and Finance
- Capital One: Leverages AI to deliver personalized financial guidance and banking solutions, adjusting credit card offers, loan choices, and savings strategies to match individual financial habits.
- Robinhood: Offers customized investment guidance and portfolio suggestions based on the user’s investment behavior and risk appetite.
Automotive
- Tesla: Although Tesla is mainly recognized for its electric vehicles, the company incorporates AI technology in its cars to understand driver preferences, such as seat adjustments, climate settings, and driving habits, creating a tailored driving experience.
Marketing
- HubSpot: Provides AI solutions for marketers to customize email marketing efforts, website content, and sales communications by analyzing customer data and behaviors.
Education
- Knewton: A dynamic learning platform that customizes educational materials to align with each student’s unique learning speed and style, making real-time adjustments based on their performance.
Smart Homes
- Google Nest: Tailors home automation features according to your daily habits, such as modifying the thermostat or lighting to suit your preferences at various times throughout the day.
AI Personalized Intelligence is increasingly essential for delivering tailored experiences, enhancing user satisfaction, and fostering business success across various sectors. Nonetheless, these examples highlight the critical need to address privacy and ethical issues as AI increasingly engages with personal data.
Your time is valuable. Just contact us or book an appointment and we will tell you what your AI Personalized Intelligence options are.
Revo DCS wan’ts to ensure the process is simple, easy to understand, accessible and efficient for all.
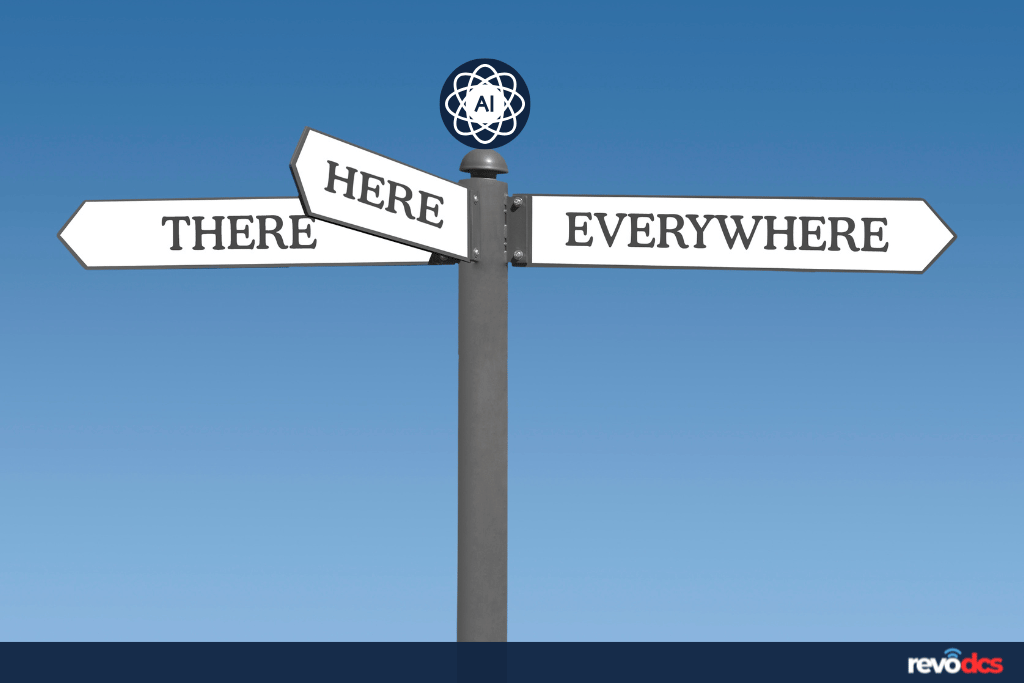
AI Personalized Intelligence In Customer Service
AI has significantly transformed customer service by improving efficiency, personalizing interactions, and enhancing customer satisfaction. Here’s how AI is being utilized in this domain:
1. Chatbots and Virtual Assistants
- 24/7 Availability: AI chatbots provide instant customer support around the clock, handling inquiries, providing information, and even resolving simple issues without human intervention.
- Multilingual Support: AI can communicate in multiple languages, broadening the customer base and improving service in diverse markets.
2. Personalization
- Customer Recognition: AI can recognize returning customers across different channels, recalling past interactions to provide a more personalized service experience.
- Tailored Responses: Based on customer history, preferences, or current context, AI can tailor responses for better relevance and engagement.
3. Automation of Routine Tasks
- Ticket Routing: AI can categorize and route customer queries to the appropriate department or agent based on the nature of the request, speeding up resolution times.
- FAQ Handling: Automatically answering frequently asked questions or providing information like opening hours, return policies, etc.
4. Predictive Support
- Proactive Outreach: By analyzing customer data, AI can predict when a customer might need assistance and reach out proactively, addressing issues before they escalate.
- Preventive Maintenance: In service scenarios, AI might predict when a product might fail or need maintenance, offering timely support or advice.
5. Sentiment Analysis
- Emotional Intelligence: AI can analyze customer sentiment through text or speech, adjusting responses to better match the customer’s emotional state, whether they’re frustrated, happy, or neutral.
- Feedback Analysis: Collecting and analyzing feedback to improve products or services continuously.
6. Voice Recognition and IVR Systems
- Natural Language Processing (NLP): Enhances voice-based systems to understand and respond to human speech more naturally, making phone support more efficient.
- Interactive Voice Response (IVR): AI can make IVR systems smarter, allowing for more natural conversation flow and quicker resolution paths.
7. Self-Service Options
- Smart Knowledge Bases: AI can help in creating dynamic, searchable knowledge bases where customers can find answers to their queries, reducing the need for direct support.
8. Customer Insights and Analytics
- Behavioral Analysis: AI provides insights into customer behavior and preferences, allowing for better service strategies and personalization.
- Churn Prediction: Identifying customers at risk of leaving, so customer service can take action to retain them.
9. Training and Quality Assurance
- AI for Coaching: AI can review customer interactions to provide feedback to human agents, helping improve service quality.
- Quality Monitoring: Automating part of quality assurance by checking for compliance, tone, and resolution effectiveness in customer interactions.
10. Omnichannel Experience
- Unified Customer Journey: AI helps in providing a seamless experience across different contact points (email, chat, social media, phone), ensuring consistency and continuity in customer service. UCaaS and CCaaS voice systems are empowering businesses of all sizes to function like fortune 500 companies.
Ethical and Privacy Considerations:
- Handling customer data with AI raises privacy concerns. Compliance with data protection laws like GDPR is crucial, as is transparency about how AI uses customer data.
Challenges:
- Balancing automation with the human touch to maintain empathy in customer interactions.
- Ensuring AI systems do not perpetuate biases or provide inaccurate information.
AI in customer service is about augmenting human capabilities, not replacing them. The goal is to make support more accessible, efficient, and personalized, ultimately enhancing customer satisfaction and loyalty. However, the human element remains vital for complex issues or where emotional intelligence is needed.
AI Personalized Intelligence Sentiment Analysis
AI sentiment analysis is a technique within natural language processing (NLP) and machine learning where AI systems interpret and classify emotions within text or speech data. Here’s how it works and its applications:
How AI Sentiment Analysis Works:
- Text Preprocessing:
- Cleaning text by removing noise like punctuation, special characters, or converting all text to lower case.
- Tokenization, where text is broken down into words or phrases (tokens).
- Feature Extraction:
- Using techniques like bag-of-words, n-grams, or more advanced methods like word embeddings (e.g., Word2Vec, GloVe) to represent text in a way that AI can understand.
- Modeling:
- Training machine learning models on labeled data to classify sentiment. Common models include:
- Supervised Learning: Algorithms like Naive Bayes, Support Vector Machines (SVM), or Deep Learning models (e.g., CNNs, RNNs, LSTMs).
- Unsupervised Learning: Lexicon-based approaches where sentiment is determined by the presence of words known to have positive or negative connotations.
- Hybrid Approaches: Combining rule-based methods with machine learning for more nuanced analysis.
- Training machine learning models on labeled data to classify sentiment. Common models include:
- Sentiment Classification:
- Outputs typically categorize text as positive, negative, or neutral. More advanced systems might detect emotions like joy, anger, sadness, etc., or even fine-grained sentiment scores.
Applications of AI Sentiment Analysis in regards to AI Personalized Intelligence:
- Brand Monitoring: Companies use it to track brand sentiment on social media, review sites, or news articles to gauge public perception and react to feedback.
- Customer Service: Analyzing customer feedback or interactions to understand customer satisfaction, tailor responses, or prioritize issues based on sentiment intensity.
- Market Research: Understanding consumer sentiment towards products, services, or market trends to inform marketing strategies or product development.
- Financial Markets: Sentiment analysis of news, social media posts, or financial reports can influence stock market predictions or investment decisions.
- Political Campaigns: Analyzing public sentiment towards policies, candidates, or political events to adjust campaign strategies.
- Healthcare: Assessing patient sentiment in feedback or medical records to improve care or detect mental health issues.
- Entertainment: Gauging audience reactions to movies, TV shows, or music to predict success or inform content creation.
- Human Resources: Analyzing employee feedback or social media to assess workplace happiness, detect potential issues, or improve company culture.
Challenges:
- Context Understanding: Sarcasm, idioms, or cultural nuances can be difficult for AI to interpret correctly.
- Language Evolution: Slang, new words, or shifts in language use can outpace AI models’ understanding unless they’re continually updated.
- Bias: Models can inherit biases from training data, leading to skewed or unfair sentiment analysis.
- Multilingual Analysis: Sentiment can vary significantly across languages and cultures, which complicates analysis in a global context.
- Privacy Concerns: Analyzing social media or public sentiment data raises ethical questions about privacy and consent.
Future Directions:
- Emotion AI: Moving beyond simple sentiment to detect and react to a broader spectrum of human emotions.
- Real-time Analysis: Improving the speed of sentiment analysis for more dynamic applications like live customer interactions.
- Integration with Other AI Technologies: Combining sentiment analysis with speech recognition, chatbots, or visual recognition for a more holistic understanding of human behavior.
AI sentiment analysis is evolving rapidly, offering insights into human emotions and opinions at scale, but it also demands careful consideration of its ethical application, accuracy, and bias.
AI Personalized Intelligence and How AI Sentiment Analysis Works
Here’s a comprehensive breakdown of how AI, particularly focusing on sentiment analysis, works:
1. Data Collection
- Sourcing: Data can come from various places like social media posts, customer reviews, emails, forums, or any text where sentiment might be expressed.
- Data Types: Text, speech (transcribed), or even visual data (through OCR or image analysis for captions or comments).
2. Preprocessing
- Cleaning: Removes noise like unwanted characters, corrects spelling, normalizes text (e.g., converting all text to lowercase).
- Tokenization: Breaks text into individual words or tokens.
- Stop Words Removal: Eliminates common words that carry little meaning for sentiment analysis (e.g., “the”, “is”, “and”).
3. Feature Extraction
- Bag-of-Words: Represents text as the frequency of each word, ignoring grammar and order.
- N-grams: Considers sequences of n items from text (e.g., bigrams for two-word phrases).
- Word Embeddings: Maps words to vectors in a high-dimensional space, capturing semantic relationships (e.g., Word2Vec, GloVe).
- TF-IDF (Term Frequency-Inverse Document Frequency): Weighs words based on how often they appear in a document versus the entire corpus, highlighting unique terms.
4. Model Training
- Supervised Learning:
- Labeling: Requires labeled data where sentiments are manually annotated as positive, negative, or neutral.
- Algorithms:
- Naive Bayes: Simple probabilistic classifier often used as a baseline.
- Support Vector Machines (SVM): Effective for high-dimensional spaces.
- Neural Networks: Including RNNs, LSTMs, or CNNs for capturing context or sequence in text.
- Unsupervised Learning:
- Lexicon-Based Methods: Uses predefined lists of words with associated sentiment scores. The sentiment of a text is then calculated based on the sum of word scores.
- Hybrid Methods: Combining rule-based systems with machine learning for better accuracy.
5. Sentiment Prediction
- Classification: The model predicts whether the sentiment of new text is positive, negative, or neutral. Some models might also predict intensity or specific emotions.
- Scoring: Rather than binary or ternary classification, some systems output a continuous score representing sentiment strength.
6. Post-Processing
- Contextual Adjustment: Adjusting predictions based on context or known issues like sarcasm.
- Aggregation: If analyzing multiple pieces of content, aggregating sentiments to provide an overall sentiment score or trend.
7. Application
- Real-time Analysis: For live applications like customer service bots or monitoring social media.
- Batch Processing: For large datasets where immediate response isn’t necessary, like in market research or brand monitoring.
Key Technologies:
- Natural Language Processing (NLP): The backbone of text understanding, including tokenization, parsing, and semantic analysis.
- Machine Learning (ML): Algorithms that learn from data to make predictions or decisions.
- Deep Learning: Neural networks that can capture complex patterns in data, especially useful for understanding context or sequences in text.
Challenges:
- Ambiguity: Words can have different meanings based on context.
- Cultural and Linguistic Nuances: Sentiment can vary widely across cultures or languages.
- Evolving Language: New slang, idioms, or shifts in language use can quickly date models.
Ethical Considerations:
- Bias: Ensuring models are trained on diverse, representative data to avoid perpetuating societal biases.
- Privacy: Handling and analyzing personal data with respect to privacy laws and ethical standards.
The process is iterative, with models often being retrained or fine-tuned as new data comes in or as the language and user behavior evolve. Each step in this pipeline can be customized or expanded based on the specific application or domain of sentiment analysis.
AI Personalized Intelligence And Security
The security of AI Personalized Intelligence (AI PI) involves several dimensions, including data privacy, system integrity, and protection against adversarial attacks. Here’s an overview of the security concerns and measures:
Security Concerns:
- Data Privacy:
- Data Collection: AI PI systems require vast amounts of personal data to function effectively, which raises concerns about unauthorized access or misuse.
- Data Breaches: With large datasets, the risk of data breaches increases, potentially exposing sensitive personal information.
- Bias and Fairness:
- AI systems can perpetuate or even amplify biases present in training data, leading to discriminatory outcomes.
- Adversarial Attacks:
- Manipulation: AI models can be tricked through adversarial inputs, leading to incorrect outputs or decisions.
- Model Poisoning: Malicious actors might tamper with training data to skew AI behavior.
- Transparency and Control:
- Users often have limited transparency into how their data is used or how decisions are made by AI, which can lead to a lack of control over personal information.
- Model Theft:
- Proprietary AI models can be targets for theft, where competitors or malicious entities attempt to reverse-engineer or steal the model.
Security Measures:
- Data Protection:
- Encryption: Encrypting data both at rest and in transit.
- Anonymization: Techniques to strip personal identifiers from data or use aggregated data where possible.
- Access Control: Strict policies on who can access what data within an organization.
- Secure AI Development Practices:
- Data Validation: Ensuring the integrity of training data to prevent poisoning attacks.
- Regular Audits: Continuous monitoring and auditing of AI models for biases or anomalies.
- Privacy by Design:
- Incorporating privacy considerations into the design of AI systems from the outset, ensuring minimal data collection, and providing users control over their data.
- Adversarial Defense:
- Robustness Testing: Training models with adversarial examples to improve resilience.
- Input Sanitization: Checking and cleaning inputs to prevent adversarial attacks.
- Regulatory Compliance:
- Adhering to data protection laws like GDPR, CCPA, which mandate certain security and privacy practices.
- AI Ethics and Governance:
- Establishing ethical guidelines for AI use, ensuring transparency, fairness, and accountability.
- User Control and Transparency:
- Providing clear explanations of how AI uses data and decisions are made, along with user options to opt-out or delete their data.
- Secure Model Deployment:
- Using secure environments like data enclaves or employing federated learning where data doesn’t need to leave the user’s device.
Challenges:
- Balancing Personalization with Privacy: The more personalized an AI system becomes, the more data it requires, potentially increasing privacy risks.
- Evolving Threats: As AI technology advances, so do the methods to exploit it.
- Scalability vs. Security: Ensuring security measures don’t hinder the performance or scalability of AI systems.
AI Personalized Intelligence Conclusion:
AI Personalized Intelligence isn’t inherently insecure, but its security depends on how it’s designed, implemented, and managed. Companies and developers are increasingly aware of these issues, leading to advancements in privacy-preserving AI techniques, like differential privacy, federated learning, and homomorphic encryption. However, the field is still evolving, and constant vigilance is required to address both current and emerging security challenges. Users should also be proactive about understanding the privacy policies of AI systems they interact with and exercising their rights under data protection regulations.
For more detailed insights, one might look into resources from organizations like OWASP for AI security guides or industry reports from tech companies on AI security practices.
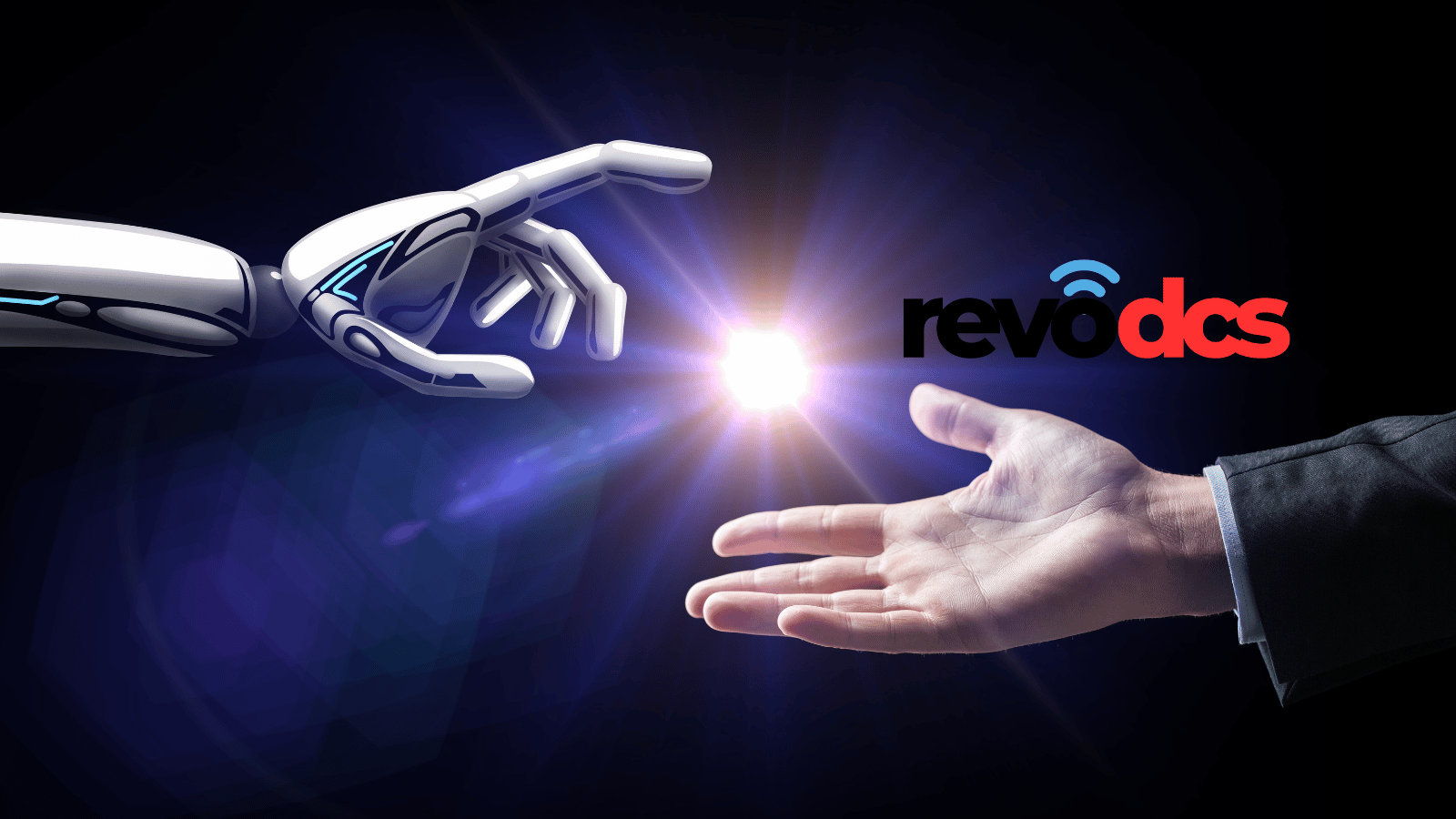
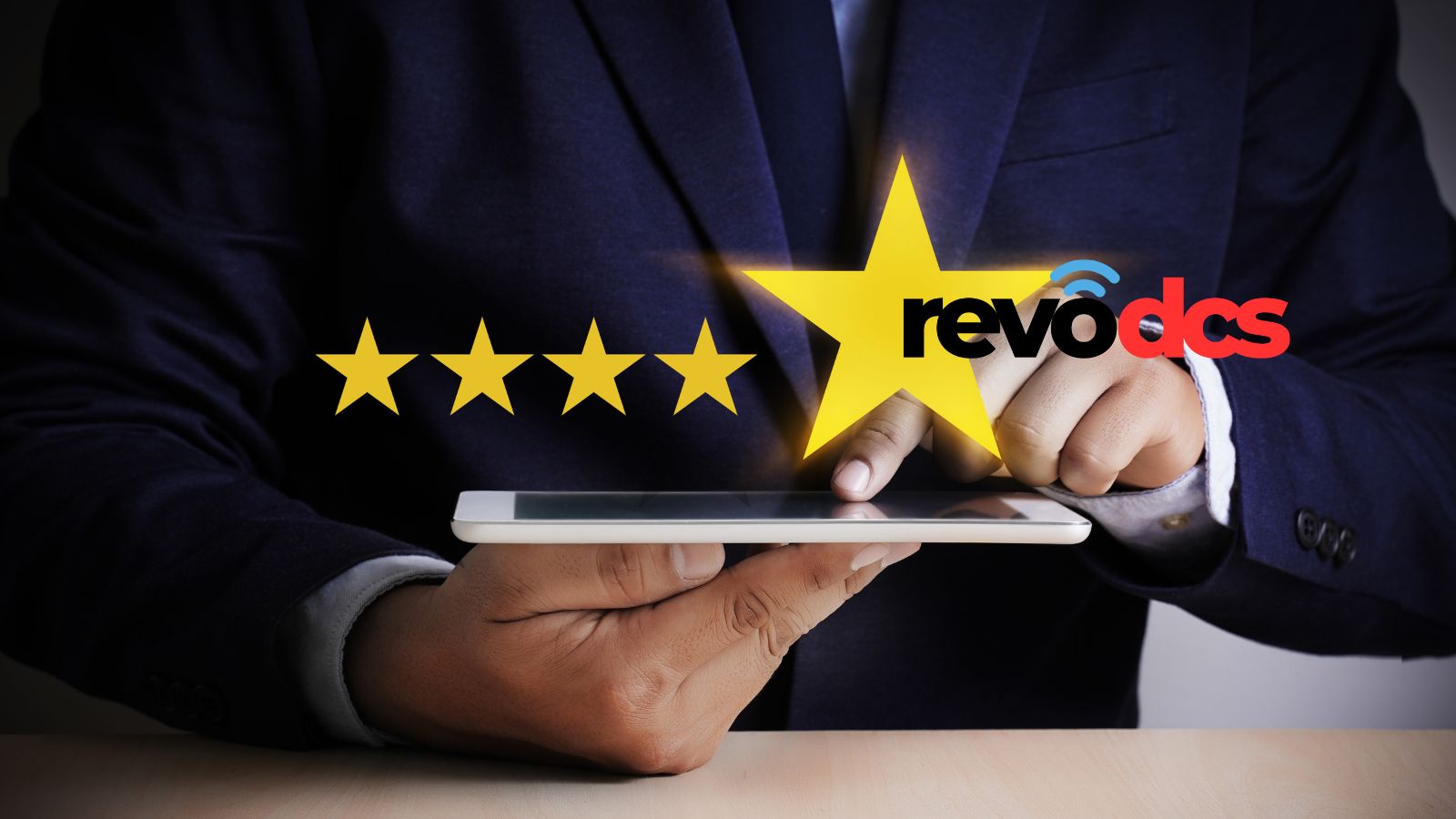
AI Personalized Intelligence And Privacy
Privacy in AI Personalized Intelligence (AI PI) is a critical concern that intersects with legal, ethical, and technical dimensions. Here’s a comprehensive look at the topic:
Key Privacy Concerns:
- Data Collection:
- Volume and Depth: AI PI systems require extensive data to personalize effectively, leading to concerns about how much personal information is collected.
- Data Types: From browsing habits to location data, voice, and biometric information, the breadth of data can be intrusive.
- Data Use and Sharing:
- Secondary Use: Data collected for one purpose might be used for others without clear user consent.
- Third-Party Sharing: Data might be shared with or sold to third parties, potentially for purposes the user was not aware of.
- User Tracking:
- Continuous surveillance or tracking of users’ activities across devices and platforms can lead to a detailed, potentially invasive, profile.
- Consent and Control:
- Informed Consent: Users might not fully understand what they’re consenting to due to complex privacy policies.
- Control Over Data: Limited options for users to control, modify, or delete their data.
- Anonymization and De-identification:
- Even anonymized data can sometimes be re-identified, especially with the use of multiple data points.
- Algorithmic Transparency:
- Lack of insight into how algorithms use personal data to make decisions can lead to a trust deficit.
Approaches to Enhancing Privacy in AI Personalized Intelligence (AI PI):
- Privacy by Design:
- Integrate privacy considerations into the system from the outset. This includes minimizing data collection, ensuring data is only used for the intended purpose, and providing user control over data.
- Data Minimization:
- Collect only the data necessary for the service, limiting the scope of personal information held by the system.
- Differential Privacy:
- Add noise to datasets to protect individual privacy while still allowing for useful aggregate data analysis.
- Federated Learning:
- Train AI models on user devices without centralizing the data, thus personalizing experiences without collecting data in one place.
- Encryption:
- Use end-to-end encryption for data in transit and at rest, with homomorphic encryption allowing computations on encrypted data.
- User Consent and Transparency:
- Clear, simple privacy policies and mechanisms for users to understand and control their data.
- Pseudonymization:
- Replace private identifiers in data with pseudonyms, making re-identification more difficult.
- Secure Multi-Party Computation:
- Allows parties to jointly compute a function over their inputs while keeping those inputs private.
- Ethical AI Frameworks:
- Develop and adhere to ethical guidelines that prioritize privacy, fairness, and accountability.
Legal and Regulatory Compliance:
- GDPR (EU): General Data Protection Regulation sets stringent rules on data handling, consent, and user rights.
- CCPA (California): California Consumer Privacy Act provides residents with rights over their personal data.
- Other Regional Laws: Many countries are following with their own data protection regulations.
Challenges:
- Balancing Personalization with Privacy: The more personalized the service, the more data it might need, which conflicts with privacy goals.
- Technological Complexity: Implementing privacy-preserving technologies can be technically challenging and resource-intensive.
- User Education: Ensuring users understand privacy settings and implications can be difficult.
- Evolving Threats: As AI techniques evolve, so do methods to exploit personal data.
Future Outlook:
- Regulatory Evolution: More jurisdictions are likely to enact or strengthen privacy laws, potentially leading to global standards.
- Privacy-Enhancing Technologies: Continued development in areas like federated learning, differential privacy, and secure computation will become more mainstream.
- User Empowerment: Tools and interfaces that make privacy management easier for users will become more prevalent.
- Ethical AI: A stronger focus on ethical AI practices where privacy is a core component of system design.
Addressing privacy in AI Personalized Intelligence is an ongoing process that requires a collaborative effort from developers, regulators, businesses, and users to ensure that personalization does not come at the expense of privacy.
AI Personalized Intelligence and AI Data Minimization Techniques
Data minimization is a principle aimed at collecting, processing, and storing only the personal data that is strictly necessary for the specific purpose it’s being used for. Here are several techniques to achieve data minimization in the context of AI, software development, and data management:
1. Purpose Limitation
- Clearly Define Purposes: Collect data only for explicit, legitimate purposes. If data isn’t needed for that purpose, don’t collect it.
- Purpose Limitation Clauses: In privacy policies, specify what data is used for what purpose, ensuring no unnecessary data collection occurs.
2. Data Collection Minimization
- Necessary Data Only: Only ask for data that’s essential for the service or feature. For instance, if an age range suffices, don’t ask for exact birth dates.
- User Input Design: Design forms or interfaces to minimize the amount of data users need to enter, using defaults or pre-filled data where appropriate.
3. Data Retention Policies
- Retention Periods: Define and enforce how long data is kept. Data should be deleted or anonymized once it’s no longer needed for the purpose it was collected.
- Automated Deletion: Implement systems that automatically purge or anonymize data after a set period unless there’s a legal or operational need to retain it.
4. Anonymization and Pseudonymization
- Anonymization: Remove or alter personal identifiers so that the data can no longer be associated with a specific person. Techniques include data masking, generalization, or perturbation.
- Pseudonymization: Replace private identifiers with fake identifiers or pseudonyms. This allows for data processing while reducing privacy risks, provided the key linking the pseudonym to real data is secured.
5. Data Aggregation
- Use Aggregate Data: When personal data isn’t necessary, use aggregated data instead. This can provide insights without exposing individual data points.
6. Privacy by Design
- Embed Privacy: From the outset of product design, consider how to minimize data use. This could mean designing systems to work with less data or to process data in ways that don’t require storage.
- Privacy Impact Assessments (PIAs): Conduct these to evaluate and minimize privacy risks in new projects or data processes.
7. Dynamic Consent
- Granular Consent: Allow users to consent to specific uses of their data rather than a blanket consent. This means collecting data only for consented purposes.
- Consent Management: Systems that let users easily manage their consents, including the ability to withdraw consent for specific data uses.
8. Data Quality
- Accuracy and Relevance: Ensure the data collected is accurate and relevant to the purpose. Poor quality or irrelevant data can lead to over-collection.
9. Technical Measures
- Encryption: Use encryption to protect data, which also supports data minimization by reducing the risk associated with data storage.
- Access Controls: Limit who within an organization can access personal data to those who need it for their work.
10. Regular Audits
- Review Data Practices: Periodically check what data is being collected, how it’s being used, and if all of it is still necessary, leading to ongoing minimization efforts.
Challenges:
- Balancing Functionality with Privacy: Ensuring services remain useful without excessive data collection.
- Regulatory Compliance: Adhering to different data protection laws which might have varying requirements for data minimization.
- User Experience: Simplifying data collection must not overly complicate or frustrate user interaction.
Tools and Technologies:
- Data Anonymization Software: Tools like ARX, k-anonymity, or differential privacy mechanisms for data protection.
- Consent Management Platforms: Solutions for managing user consents in line with privacy laws.
- Privacy-Enhancing Technologies (PETs): Including secure multi-party computation or homomorphic encryption for processing data without exposing it.
Data minimization techniques are not just about reducing data for privacy’s sake but also about enhancing security, reducing storage costs, and maintaining trust with users. It’s an evolving practice as technology and regulations advance.
Based on very expensive studies on the topic, we see that 70% of companies will adopt AI in some form or fashion by 2030. In 2025, we are already hearing about it everywhere. 2025 will be the year that AI will sky rocket to be the magic behind approximately 95% of customer interactions in regards to the Customer Experience.
Based on the data, we believe that AI will power 95% of customer interactions by the end of 2025, and 19 in every 20 customer interactions will be AI-assisted in 2025.
Book An Appointment To Learn More
AI Personalized Intelligence for CCaaS Voice Systems
CCaas, Contact Center as a Service is a cloud based call center solution that can utilize artificial intelligence to improve customer service and operational efficiency. AI-powered CCaaS can include chatbots, routing, analytics, and automation
The benefits of CCaaS with AI include:
• Shorter call times and shorter hold times
• Greater productivity
• Greater insights into customer needs
• Increased sales and profits
• Better customer interactions
• Better time management
AI Personalized Intelligence and AI Voice Agents
Either in front of a UCaaS voice system, or even as a primary voice solution for all types of businesses, an automated conversational AI Voice Solution will in fact go a long way.
Here are some basic features that many small and medium businesses are considering as we speak:
• Answer ALL inbound calls instead of missing calls or sending callers to voicemail.
• Free up employee resources to focus on work and allow the system to manage intake, FAQ’s, etc.
• Create 24/7/365 availability for your company and so much more!
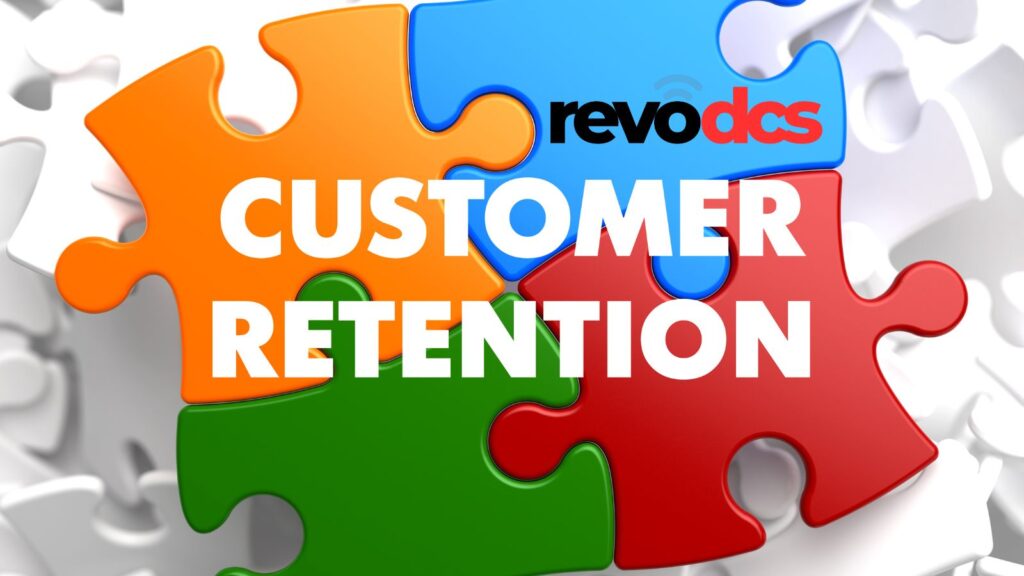
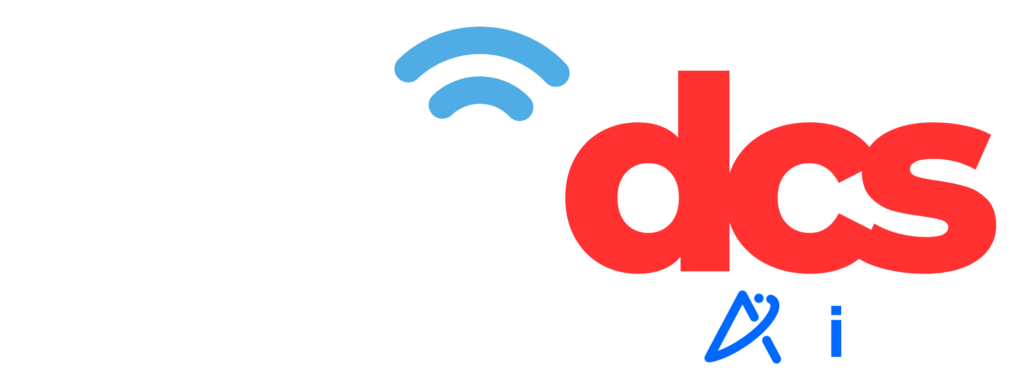